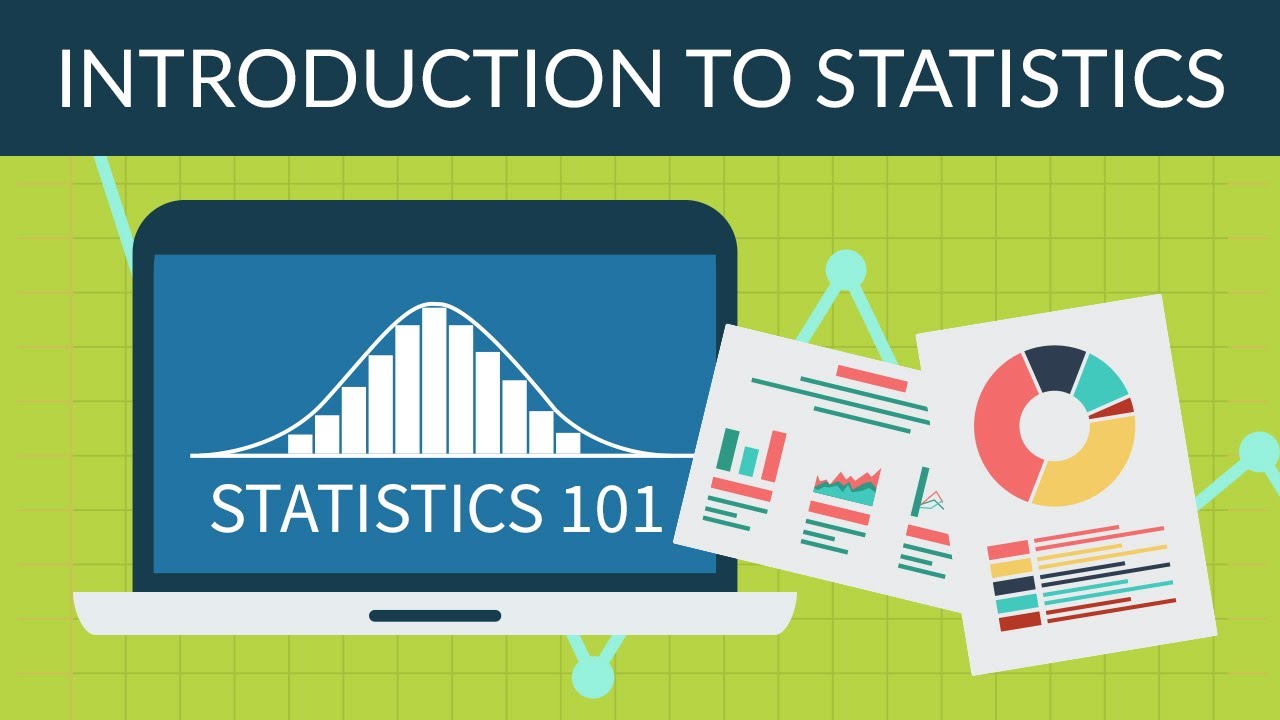
Introduction to Statistics for Data Science
Published Date: Aug. 29, 2024
Statistics is a branch of mathematics that involves collecting, analysing, interpreting, and presenting data. It provides tools and methods to understand and make sense of large amounts of data and to draw conclusions and make decisions based on the data.
In practice, statistics is used in a wide range of fields, such as business, economics, social sciences, medicine, and engineering. It is used to conduct research studies, analyse market trends, evaluate the effectiveness of treatments and interventions, and make forecasts and predictions.
Examples:
- Business - Data Analysis(Identifying customer behavior) and Demand Forecasting
- Medical - Identify efficacy of new medicines(Clinical trials), Identifying risk factor for diseases(Epidemiology)
- Government & Politics - Conducting surveys, Polling
- Environmental Science - Climate research
Types of Statistics:
1. Descriptive Statistics: Descriptive statistics deals with the collection, organization, analysis, interpretation, and presentation of data. It focuses on summarizing and describing the main features of a set of data, without making inferences or predictions about the larger population.
2. Inferential Statistics: Inferential statistics deals with making conclusions and predictions about a population based on a sample. It involves the use of probability theory to estimate the likelihood of certain events occurring, hypothesis testing to determine if a certain claim about a population is supported by the data, and regression analysis to examine the relationships between variables.
Population Vs Sample
Population refers to the entire group of individuals or objects that we are interested in studying. It is the complete set of observations that we want to make inferences about. For example, the population might be all the students in a particular school or all the cars in a particular city.
A sample, on the other hand, is a subset of the population. It is a smaller group of individuals or objects that we select from the population to study. Samples are used to estimate characteristics of the population, such as the mean or the proportion with a certain attribute. For example, we might randomly select 100 students.
Examples
1. All cricket fans vs fans who were present in the stadium
2. All students vs who visit college for lectures
Things to be careful about which creating samples
1. Sample Size
2. Random
3. Representative
Parameter Vs Statistics
A parameter is a characteristic of a population, while a statistic is a characteristic of a sample. Parameters are generally unknown and are estimated using statistics. The goal of statistical inference is to use the information obtained from the sample to make inferences about the population parameters.
Inferential Statistics
Inferential statistics is a branch of statistics that deals with making inferences or predictions about a larger population based on a sample of data. It involves using statistical techniques to test hypotheses and draw conclusions from data. Some of the topics that come under inferential statistics are:
- Hypothesis testing: This involves testing a hypothesis about a population parameter based on a sample of data. For example, testing whether the mean height of a population is different from a given value.
- Confidence intervals: This involves estimating the range of values that a population parameter could take based on a sample of data. For example, estimating the population mean height within a given confidence level.
- Analysis of variance (ANOVA): This involves comparing means across multiple groups to determine if there are any significant differences. For example, comparing the mean height of individuals from different regions.
- Regression analysis: This involves modelling the relationship between a dependent variable and one or more independent variables. For example, predicting the sales of a product based on advertising expenditure.
- Chi-square tests: This involves testing the independence or association between two categorical variables. For example, testing whether gender and occupation are independent variables.
- Sampling techniques: This involves ensuring that the sample of data is representative of the population. For example, using random sampling to select individuals from a population.
- Bayesian statistics: This is an alternative approach to statistical inference that involves updating beliefs about the probability of an event based on new evidence. For example, updating the probability of a disease given a positive test result.